Why Predictive Maintenance Still Struggles at Scale
As modern manufacturing environments scale, they face several challenges in predictive maintenance such as:
- Many manufacturers find it hard to move beyond basic monitoring to truly predictive insights that scale across complex, high-output environments.
- Real-time sensor data, maintenance logs and telemetry pour in from every machine, making it overwhelming to extract actionable signals without the right AI systems in place.
- Legacy platforms and siloed data make it nearly impossible to unify equipment records and spot early signs of failure before it's too late.
- Manually identifying patterns in fragmented datasets across locations is time-consuming, error-prone and doesn't scale with modern production demands.
Why AI is the Next Step in Industrial Maintenance
Traditional predictive maintenance tools rely on fixed rules or basic threshold alerts. They’re rigid, often inaccurate and require manual configuration. AI can:
- Ingest and analyse both structured (sensor logs, telemetry) and unstructured data (technician notes, manuals)
- Identify subtle failure patterns that are invisible to human analysts
- Continuously learn and adapt based on new data
- Generate real-time insights that reduce downtime and extend asset lifespan
AI-Powered Predictive Maintenance at Scale
In high-output environments, equipment failure doesn’t just disrupt workflows, it impacts revenue, safety and reputation. That’s why more enterprises are turning to AI-powered predictive maintenance. Unlike rules-based systems, AI learns from vast datasets and adapts over time. It detects patterns that hint at early-stage failures and recommends proactive actions.
For example, Shell, one of the world’s largest energy companies, has deployed AI at scale using C3 AI to oversee 10,000+ critical equipment assets. These include pumps, compressors, control valves and other high-risk components.
By leveraging advanced AI models, Shell can detect signs of equipment degradation and failure well before they escalate, allowing teams to take proactive action. This shift from reactive to predictive has helped the company significantly reduce unplanned downtime, avoid costly production interruptions and mitigate environmental and safety risks.
This was made possible through a robust AI infrastructure that:
- Ingests over 20 billion rows of data each week from more than 3 million sensors
- Trains and runs 11,000+ machine learning models continuously in production
- Generates 15 million predictive insights daily, enabling near real-time decision-making at scale
- Supports global deployment across Shell’s entire energy value chain, from upstream to downstream
Scaling Predictive Maintenance with the Right Infrastructure
Much like Shell, any large-scale manufacturing enterprise requires a robust and scalable infrastructure to power their AI initiatives, so its predictive models operate in real-time and grow alongside dynamic operational demands.
Here’s how our AI Supercloud can help manufacturing companies scale their AI projects:
-
High-Performance Compute for AI: Training and running predictive models at scale demands powerful compute. With the AI Supercloud, you gain access to the most advanced GPU Clusters for AI including NVIDIA HGX H100, NVIDIA HGX H200 and the upcoming NVIDIA Blackwell GB200 NVL72/36. These systems deliver unmatched performance for AI and high-performance computing (HPC) workloads, with the shortest delivery time in the market.
-
High-Throughput Storage for Streaming Data: Real-time analytics depends on the ability to ingest and manage continuous streams of telemetry, sensor data and system logs. Our GPU clusters for AI are equipped with NVIDIA-certified WEKA storage featuring GPUDirect Storage support, ensuring high throughput and low-latency access to vast amounts of structured and unstructured data.
-
Advanced Networking for Low-Latency Performance: To ensure low-latency data transfer across facilities, the AI Supercloud offers cutting-edge networking solutions with NVLink and NVIDIA Quantum-2 InfiniBand. These technologies significantly reduce latency and maximise bandwidth, ideal for applications that rely on rapid signal detection and response.
-
Seamless Integration with Existing Systems: Deploying large-scale AI for predictive maintenance requires seamless integration with legacy systems. The AI Supercloud ensures no vendor lock-in and full compatibility with third-party platforms. Our ecosystem includes support for Ops tools like Grafana, ArgoCD and Harbor, as well as MLOps frameworks such as Kubeflow, MLFlow, UbiOps, and Run.ai. Whatever tools your teams rely on, we help ensure a smooth, scalable deployment.
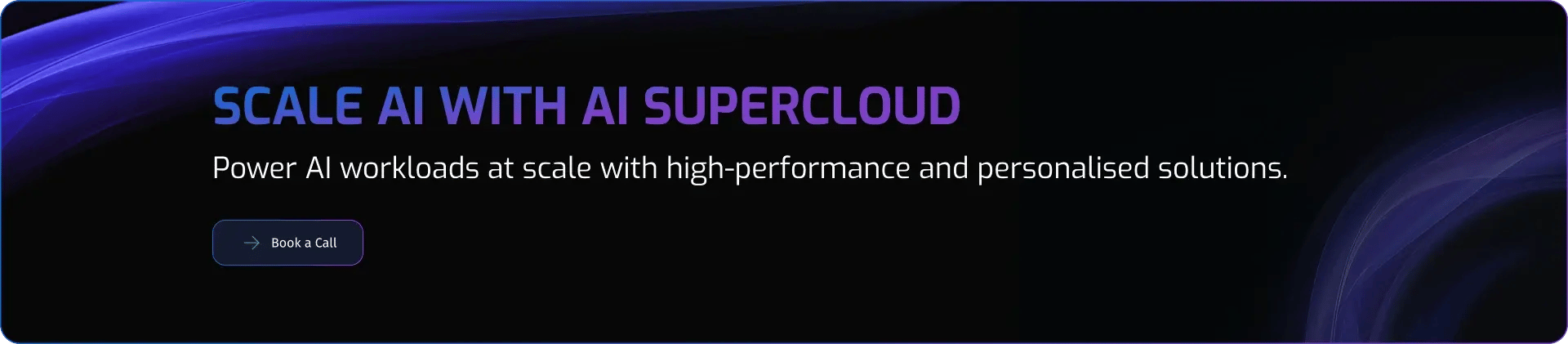
FAQs
What is AI-powered predictive maintenance?
AI-powered predictive maintenance refers to the use of AI and machine learning to detect early signs of equipment failure, allowing companies to take proactive action before breakdowns occur.
Why do traditional predictive maintenance systems fall short?
They rely on fixed rules and thresholds, struggle with large data volumes, and don’t adapt over time, making them inefficient at scale.
How does AI improve accuracy in failure detection?
AI models learn from historical and real-time data, identifying complex patterns that are often invisible to human analysts or rule-based systems.
What infrastructure is needed for AI at scale?
Scalable compute, high-throughput storage, low-latency networking, and open system integration are all essential to support real-time model training and inference.